The Power of Prediction: Harnessing Predictive Analytics for Business Transformation
In today's data-driven era, predictive analytics is leveraged to steer decision-making, enhance operational efficiencies, and unlock new revenue opportunities. By integrating historical data, statistical algorithms, and machine learning, companies can forecast future trends and predict behaviors with impressive precision. This ability is particularly transformative in multisided business platforms where data is constantly being generated across multiple customer segments and channels.
What Are Multisided Business Platforms (MSPs)?
In data monetization and predictive analytics, it is important to understand which business models are most predisposed to success. Industries with multisided business models often exhibit high levels of data liquidity due to several intrinsic factors related to the nature and dynamics of their operations. A multisided business model involves platforms or companies that facilitate interactions between two or more distinct but interdependent groups of customers or users. Examples include online marketplaces, social media platforms, and payment processing companies. Here’s why these industries typically have high data liquidity:
Diverse Interaction Points
Multisided platforms facilitate a variety of interactions among different user groups. For example, a marketplace connects buyers and sellers, a payment platform links merchants and consumers, and a social media site interacts with individuals, advertisers, and content creators. Each interaction generates data, often in large volumes, such as transaction histories, user behavior analytics, content preferences, and engagement metrics.
Continuous Data Generation
The ongoing interactions on these platforms ensure a continuous stream of data. Each transaction or engagement on the platform adds new data points, enriching the existing datasets. This continuous data flow helps maintain high data liquidity, making it readily available for analysis and monetization.
Network Effects
Multisided platforms often benefit from network effects, where the platform's value increases as more users join and interact. This increase in users and interactions boosts the volume and variety of data collected, enhancing data liquidity. As data accumulates, these platforms can leverage more detailed insights to improve user experiences, tailor services, and attract even more users, further reinforcing the network effect.
Cross-Group Data Utilization
Data from one group of users can often be leveraged to enhance the services or products offered to another group. For instance, consumer behavior data on an e-commerce platform can be analyzed to help sellers optimize their offerings or marketing strategies. This cross-utilization of data increases its value and liquidity, as it is actively being used and exchanged across the platform.
Scalability and Expansion
Multisided platforms are typically scalable, expanding rapidly into new markets or user segments. This scalability leads to more data generation and a broader base from which data can be harvested. As platforms scale, they also diversify the types of data collected, encompassing a wider array of behaviors, preferences, and interactions.
Diverse Revenue Recognition Models
The impact of using diverse revenue recognition models in a business is significant and multifaceted, affecting both financial reporting and data management. Different models can lead to variations in how revenue is recognized and reported, altering the apparent financial health of a company and influencing stakeholder decisions and investment strategies. Additionally, these models affect data accuracy and the complexity of data management, as each model requires specific data tracking and analysis to ensure compliance with regulatory standards.
In summary, the high levels of data liquidity found in industries with multisided business models are driven by the continuous, diverse, and expansive nature of the data generated through their platform interactions. This liquidity supports robust analytics and operational agility and enhances the platform’s ability to innovate and maintain a competitive edge.
.webp)
Strategic Advantages of Predictive Analytics
Predictive analytics offers several strategic advantages for multisided business models, crucial for thriving in competitive environments:
- Enhanced User Experience: By analyzing user behavior and preferences, companies can personalize experiences, recommend relevant products or services, and anticipate user needs, improving satisfaction and retention across different user groups.
- Improved Matching Accuracy: Predictive analytics can refine the algorithms used to match different sides of the platform, such as buyers and sellers or service providers and consumers. This leads to more successful interactions, enhancing the value of the platform for all participants.
- Operational Efficiency: These analytics help forecast demand and supply dynamics within the ecosystem, allowing for better resource allocation, inventory management, and capacity planning. This impacts advertising inventory, event logistics, and contract management for events and media companies.
- Strategic Decision Making: By providing insights into trends and potential future scenarios, predictive analytics aids in making informed strategic decisions, from identifying new market opportunities to adjusting pricing models dynamically.
- Risk Management: Predictive models can foresee potential risks and fraudulent activities before they impact the business, enabling proactive measures to mitigate these risks. Risk mitigation can include customer churn, program performance, contract performance, market downturn, and employee attrition.
- Monetization Strategies: Insights from data can help tailor advertising, improve monetization strategies, and optimize the effectiveness of promotional campaigns, thus increasing revenue streams.
Incorporating predictive analytics into a multisided platform can transform data into a strategic asset, driving growth and competitive advantage by aligning business operations closely with market demands and user expectations.
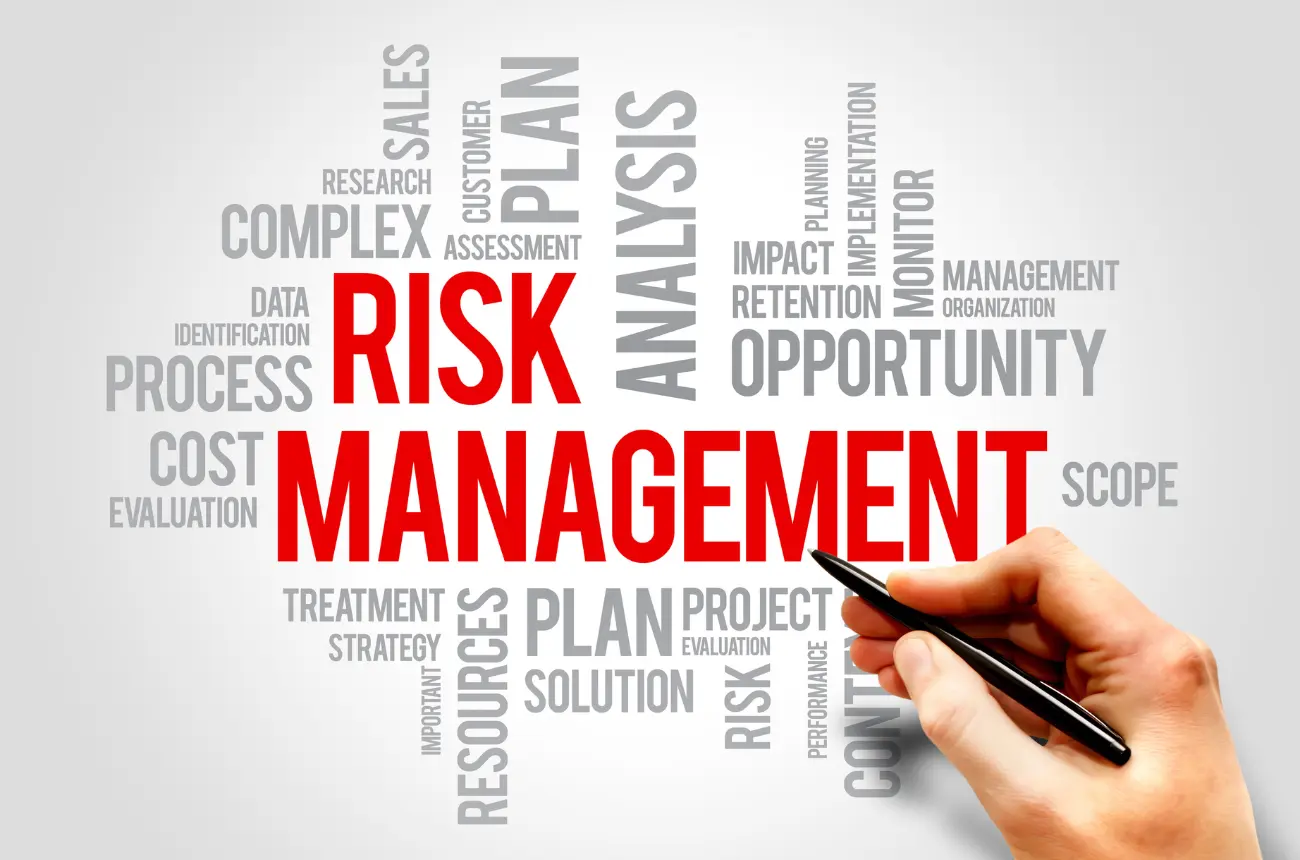
Predictive Analytics in Media: A Closer Look at Use Cases
With their rich datasets and dynamic content landscapes, media companies are ideally placed to leverage predictive analytics. Here are several impactful applications:
CHURN RISK
Advertiser Churn: By analyzing historical data, such as frequency and volume of ad buys, campaign success rates, and client feedback, predictive models can flag advertisers at risk of reducing their spend or leaving altogether.
For example, if a model detects that advertisers with decreasing engagement rates tend to churn within three months, media companies can initiate targeted retention strategies tailored to these advertisers. These strategies might include personalized offers, improved ad placement options, or custom campaign analytics reports designed to demonstrate the platform's value.
Subscriber Churn: Media businesses utilize predictive models to identify which subscribers might soon cancel their subscriptions. Insights into why subscribers leave—be it content dissatisfaction or competitive pricing—enable targeted interventions to retain them.
PRODUCT PERFORMANCE
Ad Campaign Performance: By leveraging data from past campaigns, including viewer engagement metrics, conversion rates, and demographic information, predictive models can forecast the performance of future campaigns under various scenarios. For instance, a media company might use predictive analytics to determine the optimal mix of content, audience targeting, and ad timing to enhance viewer engagement and conversion rates. Additionally, predictive models can identify which content themes or formats resonate most with specific audience segments, allowing for the customization of ads to audience preferences.
Leveraging First-Party Data: With third-party cookies phasing out, media companies increasingly rely on first-party data to fuel their predictive models. This data provides deeper insights into consumer behaviors on their platforms, enabling more accurate predictions about content preferences and advertisement placements.
Enhancing Lead Generation Campaigns: Predictive analytics can significantly improve the effectiveness of lead generation campaigns by identifying characteristics of leads that are more likely to convert. Media companies can use these insights to tailor their messaging and targeting strategies, thereby increasing their campaigns' conversion rates and ROI.
Optimizing Event Outcomes: For media companies that host webinars, conferences, or other events, predictive analytics can forecast attendee interests and engagement levels. This intelligence allows for customizing event content to match audience preferences, enhancing participant satisfaction and engagement.
CONTENT PERFORMANCE
Content Optimization: Predictive analytics guides media entities in crafting content strategies aligned with viewer preferences, ensuring resource allocation towards content with higher engagement potential. Preferences can include time, format, length, channel, and style mapped to specific themes and audience segments.
Informing Editorial Decisions: Editorial teams can benefit from predictive analytics by identifying trending topics and predicting reader interest in various subjects. This can guide content creation efforts, ensuring that resources are invested in articles and features more likely to resonate with their audience. For example, by analyzing reader reactions to different themes or writers, editorial teams can adjust their content strategies to better align with emerging trends, increasing readership and engagement.
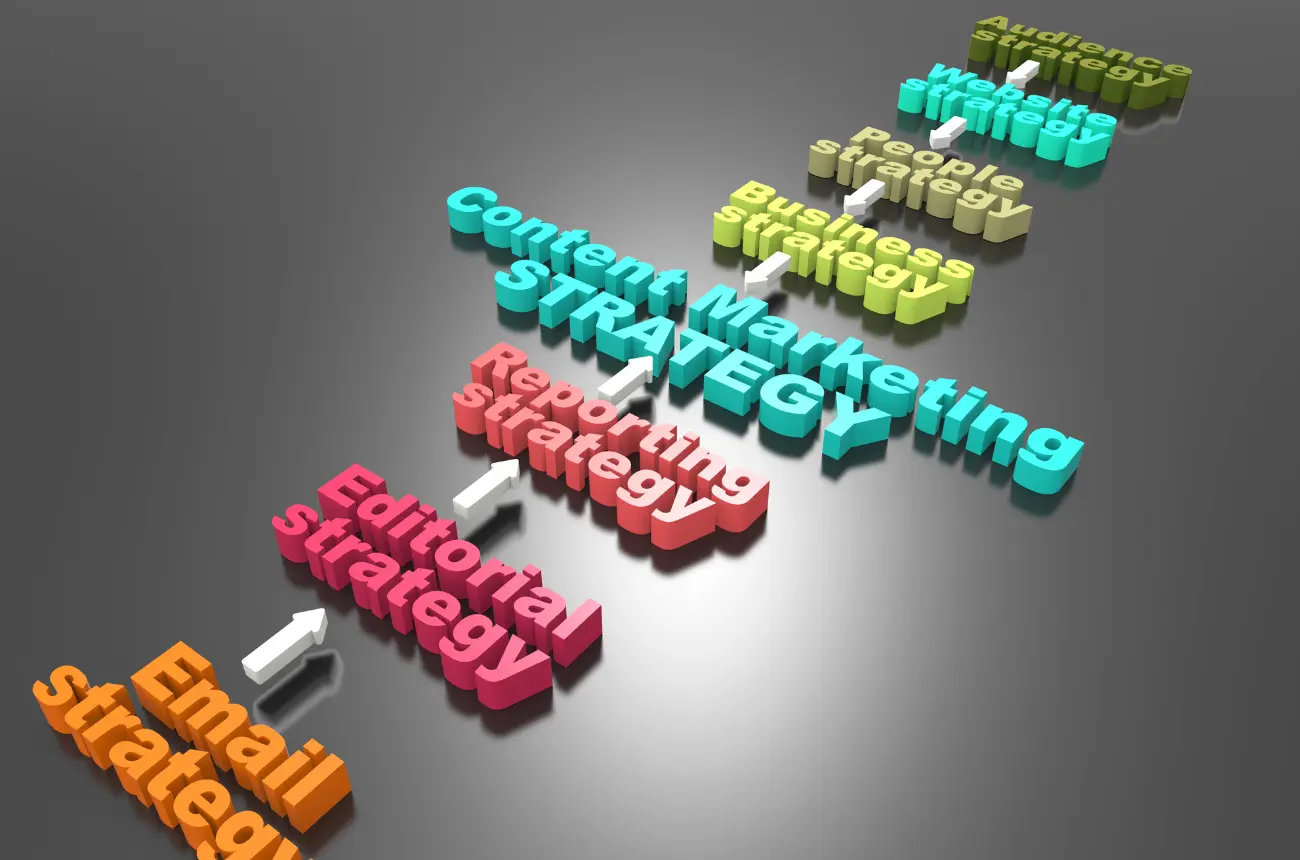
Common Pitfalls in Predictive Analytics
While predictive analytics can provide substantial benefits, several challenges may impede its successful implementation:
Data Quality and Depth: The precision of predictive analytics hinges on high-quality, comprehensive data. Inaccuracies or data gaps can lead to faulty conclusions, which might result in costly missteps.
Model Overfitting: Creating models overly tailored to historical data can fail in generalizing future conditions, leading to unexpected outcomes when applied in different contexts.
Organizational Resistance: The disruptive nature of predictive analytics might meet resistance internally, as stakeholders may prefer traditional methods over data-driven strategies.
End-to-End Workflows: Merely observing data falls short. Automated workflows are critical to act on insights effectively. These workflows ensure actions are taken, measured, and held accountable.
Regulatory Compliance: With stringent data privacy laws, companies must ensure that their predictive analytics practices comply with all regulations to avoid legal and reputational risks.
Conclusion
Predictive analytics offers businesses a powerful tool to navigate the complexities of modern markets. By turning vast data into actionable insights, companies across various sectors can remain competitive and pioneer new growth and efficiency strategies. For media companies, in particular, integrating predictive analytics into their operational and strategic frameworks is not just an option but a necessity to thrive in an increasingly digital world.
Set up a complimentary assessment call to see if predictive analytics are right for your company.
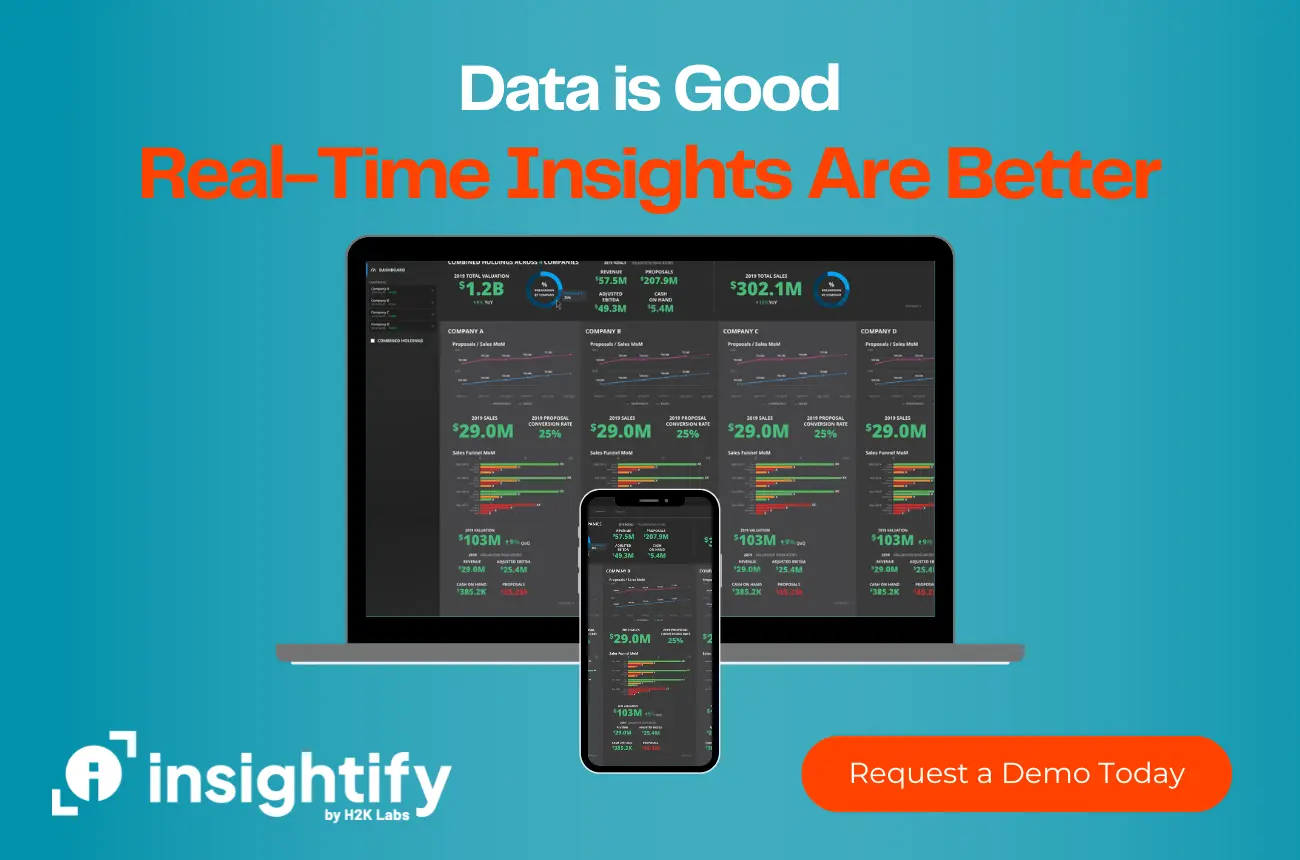